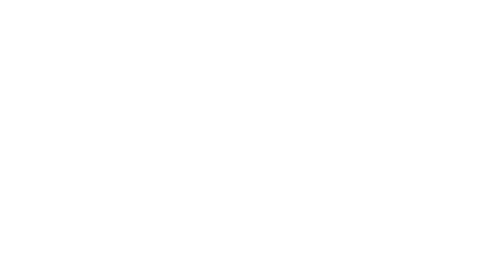
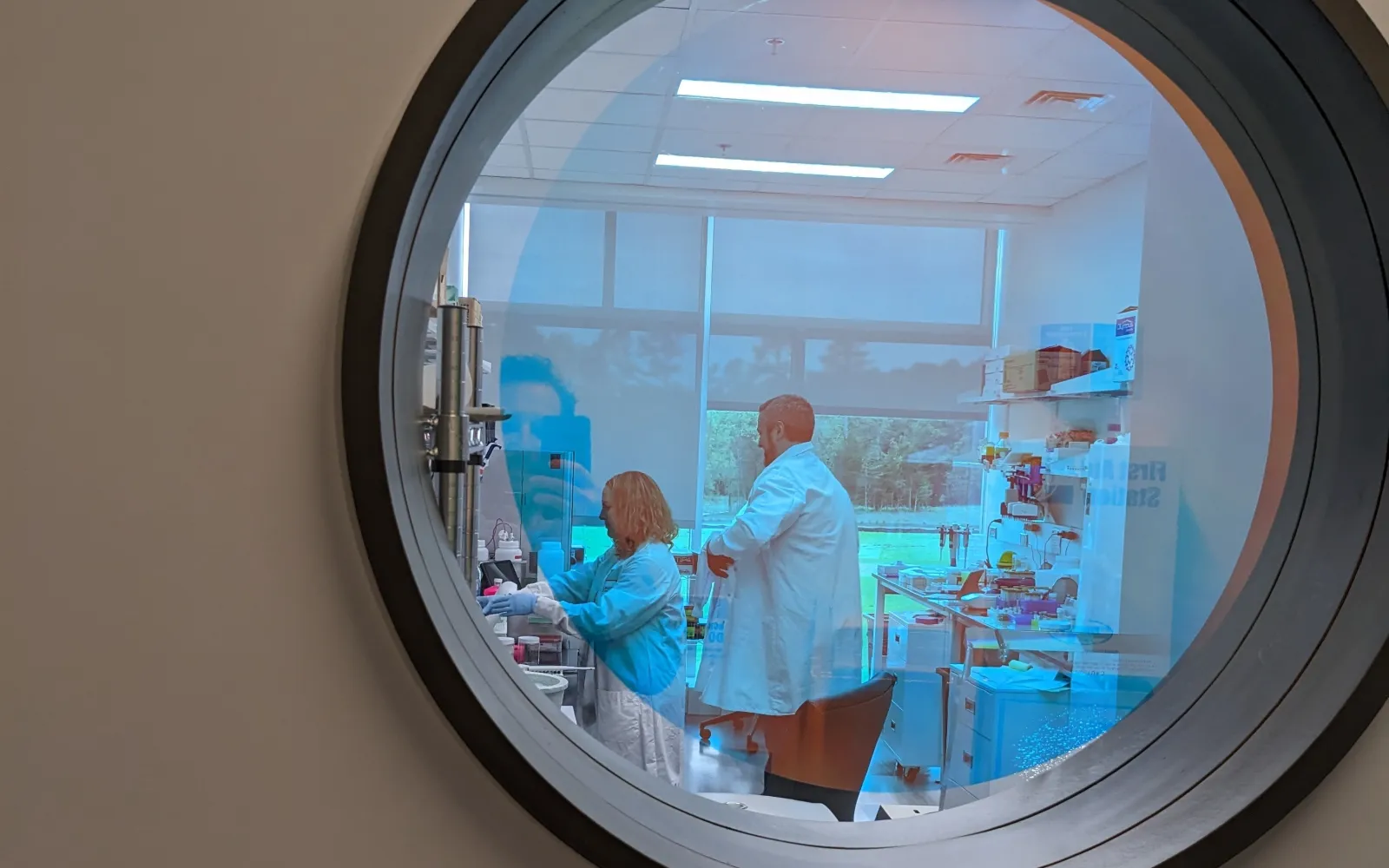
Creyon Bio
Solving the oligonucleotide drug design problem
The DCVC Deep Tech Opportunities Report (which debuted this year) summarizes our thinking about the deep tech investment areas we consider the most exciting, important, and consequential. It’s also a guide to the inspiring work innovators inside and outside the firm’s portfolio are doing to extend human capabilities, save the environment, and make everyone’s lives longer, healthier, and easier.
Three of this report’s opportunities bore on the augmentation of human abilities. What follows is a summary of one of them.
Opportunity 3.1: Predictive Drug Development
With the emergence of oligonucleotide-based medicines (OBMs), we entered the era of “information drugs” engineered to overcome specific flaws in the body’s genetic program. Once we understand how a mistake in our DNA or RNA causes a disease, we can, in principle, design an OBM to fix it. There’s just one big problem. Inside the body, many potential drug molecules do more harm than good. That’s why traditional pharma companies must sift through thousands of drug candidates, and spend months or years on toxicity studies in animals, to find just a few molecules that don’t have dangerous side effects. What’s needed is a new generation of technologies for engineering compounds that drug makers know in advance will be safe and effective, eliminating the old trial-and-error approach to drug screening and vastly speeding up safety studies and clinical trials.
DCVC Bio has invested in a collection of startups exploring new computational approaches to this problem, for both OBMs and traditional small-molecule drugs. One example is Creyon Bio, which has built a library of survey compounds representative of the kinds of nucleotide sequences and added chemical units found in OBMs. It studies how those compounds affect the livers, kidneys, cardiovascular systems, and nervous systems of mammals. At the same time, test-tube assays reveal whether the survey compounds are effective in patient cells. The result is a proprietary dataset so large that the company can use it to train machine-learning algorithms to predict whether novel OBMs will have toxic or immune-stimulating side effects.
“We’re trying to solve the oligonucleotide design problem, which is that the space of possibilities is so huge, trial and error completely fails,” says Swagatam Mukhopadhyay, Creyon’s cofounder and chief scientific officer. “We said, ‘What is the minimal set of sequences we need to study to be maximally informative about the pharmacologic design space?’ That generalization allows us to come up with molecules that are orders of magnitude better, in the sense that we have high confidence in their safety and efficacy from the get-go,” before it’s ever delivered to a patient.
“The applications of machine learning and artificial intelligence in the pharmaceutical sector are still very much in their infancy,” says DCVC Bio managing partner John Hamer. But with a rational, predictable way to design low-toxicity medicines, he says, drug makers could create treatments for the millions of Americans with rare, untreated diseases: “We call that the long tail of drug development, and no one’s touching it today.”
Read and/or download the DCVC Deep Tech Opportunities Report here.